The objective of this study was to evaluate the performance of 3 bioassessment models for reference data sets collected from the Australia Capital Territory (ACT), the Yukon River Basin (YT), and the Laurentian Great Lakes (GL) built following the standard Canadian Aquatic Biomonitoring Network (CABIN) method. To evaluate the models, we used validation reference-site data, which were artificially impaired to simulate 3 levels of eutrophication by varying the proportions of sensitive, intermediate, and tolerant taxa. Models correctly classified 56 to 62% of reference sites. Type 1 errors (assessing reference sites as degraded) were high for all data sets and ranged from 30 to 75%, in part because the biological communities of the validation sites extended to or beyond the range of the reference-site data used to build the models. Capturing the full range of ecological variation with adequate sample size is critical for reference-condition approach (RCA)-type models. Type 2 errors (assessing degraded sites as in reference condition) varied greatly among data sets and for each reference group within each data set. Resource managers must carefully consider the risks associated with making errors. Thus, standard methods for quality assurance of assessment models should include simulated data so that error rates and adjusted assessment thresholds can be reported to ensure that degradation can be detected and that undisturbed sites are not mistakenly subjected to unnecessary management action.
How to translate text using browser tools
3 October 2014
Performance of the Standard Cabin Method: Comparison of BEAST Models And Error Rates to Detect Simulated Degradation from Multiple Data Sets
Stephanie A. Strachan,
Trefor B. Reynoldson
ACCESS THE FULL ARTICLE
It is not available for individual sale.
This article is only available to subscribers.
It is not available for individual sale.
It is not available for individual sale.
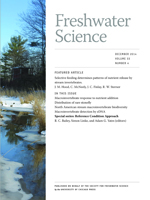
Freshwater Science
Vol. 33 • No. 4
December 2014
Vol. 33 • No. 4
December 2014
BEAST
bioassessment
CABIN
predictive modeling
reference condition approach
simulated disturbance
Type 1 error